Ping Yin
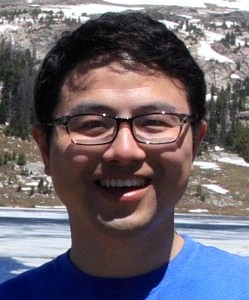
Dr.Yin is interested in Geographic Information Science (GIScience) with a focus on the development of new spatial/spatio-temporal analysis methods and applications to tackle environmental and health problems.
Early in his research career, he worked on several topics in GIScience such as spatial data interoperability, Internet-based Geographic Information Systems (GIS) services, spatio-temporal data model and 3D geovisualization. In addition, He has two-year working experience in National Geomatics Center of China in developing Client-Server and web-based GIS application systems.
His current research is focusing on health study with GIS, statistical analysis and spatial optimization techniques. For the study on disease risk modeling and social disparities in health, he used hierarchical Bayesian models to estimate the spatial-temporal patterns of lung cancer incidence by sex and race in Georgia 2000-2007 at the census tract level. The effect of area-based socioeconomic status on the lung cancer incidence risk of each subgroup is also assessed through the modeling. For health care planning, he is focusing on how to deliver better health services by optimizing the locations of health care facilities. A new locational optimization model and a new spatial demand representation approach are proposed and applied with GIS to optimally site ambulances. This research has been published in the peer-reviewed journal "Applied Geography" and reported on the website and newspaper of University of Georgia. For disease surveillance, he is working on the development of methods for detecting irregular-shaped clusters of disease in space.